OpenHard Problems in AI: A Step on the Road to AGI
By Will Glaser, CEO
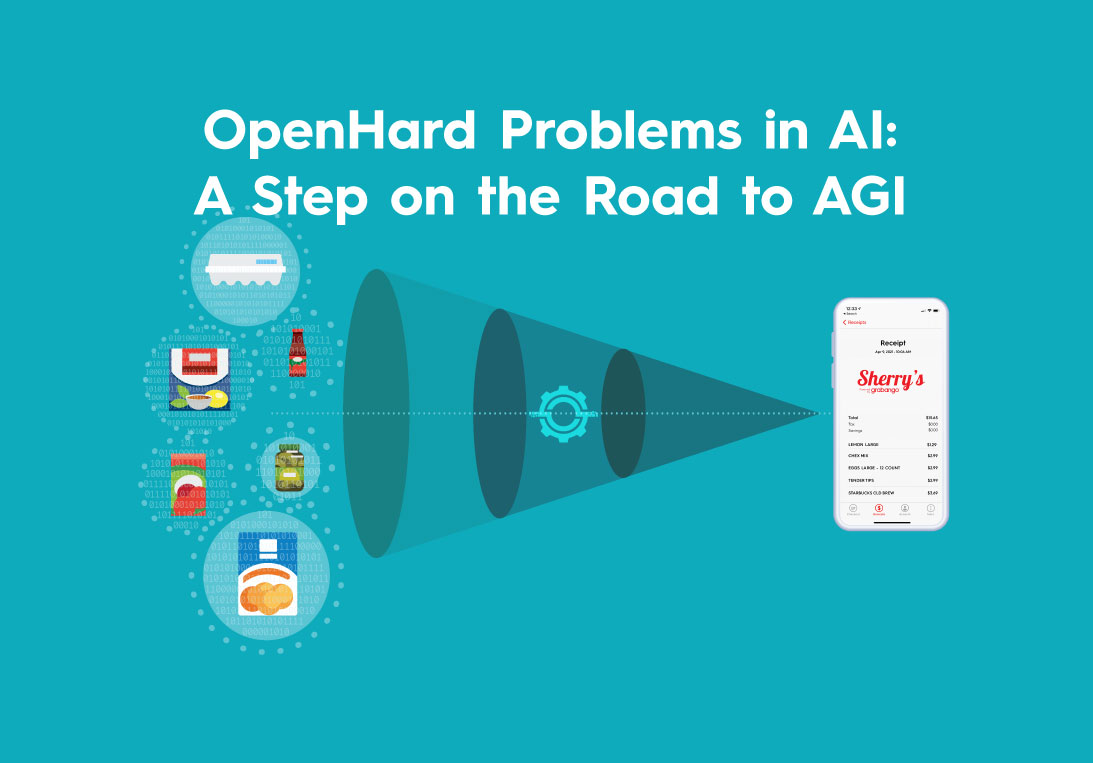
Artificial General Intelligence (AGI) is the stuff of science fiction. It refers to the ability of a computer system to reason like a person and operate effectively on the incredibly wide array of open-ended problems that we humans manage so effortlessly. AGI is a technical term describing some of our favorite characters in “Star Trek”, “2001: A Space Odyssey”, “The Terminator”, and many other popular pieces of modern fiction. While it is fun to be transported into these future worlds, computer science is a very long way from creating an AGI.
Practically speaking, the first uses of Artificial Intelligence (AI) at consumer-scale have been in one of two classes. The earliest AI successes were in narrow-domain fields like chess, go, and spell check. These systems operate on a tightly defined set of inputs and rules upon which to build an algorithmic framework. Developers mastered the challenges of these narrow domains and triumphed with superhuman performance. The second area of great success in AI has been for soft-range applications like internet search, music recommendation, and social feeds. These applications are essential in filtering the mountains of available material down to a manageable number of human-scale choices. We consider them to have a soft-range of acceptable solutions because, even with a fair proportion of false-positives and false-negatives, the filtering is still helpful. We accept their artificial judgment to curate our daily lives.
With many examples of these two classes of AI victories (narrow-domain and soft-range) solidly in hand, the new frontier of AI sees us leaving the familiarity of both areas at one time. Efforts in autonomous vehicles are addressing a problem that is simultaneously open-domain and hard-range. Not AGI for sure, but a clear step towards it. The input (domain) is open to anything a vehicle might encounter on a real roadway, including a steady flow of zero-day (unique) events that were unanticipated by developers. Further, the tolerance for error in the output (range) is essentially zero, as lives hang in the balance. From a statistical point of view, driverless cars already work pretty well most of the time. The occasional problems they face stem from the system having to manage all possible inputs, without any acceptable range for catastrophic failure. Before we reach level-5 driverless autonomy and ride in cars without a steering wheel, developers must solve this truly OpenHard problem. They must operate in a domain that is open to all input and meet a very high range of output quality to satisfy a hard measure of success.
Our work at Grabango is tackling another of these OpenHard problems: improving the retail shopping experience by eliminating lines at checkout. While this may seem like a simple application, it’s the OpenHard aspects of the problem that make it a significant challenge. In retrofitting existing stores, we’ve had to embrace the dynamic complexity of a changing product mix, out of place items, promotional displays, variable pricing, and a surprisingly diverse array of shopper behaviors. We receive these varied inputs entirely as pixels and virtually everything is an edge case. This open-domain is a part of what makes the problem so much more complex than what’s come before. The other half of the work is satisfying the hard-range, as success is measured against the unforgiving metric of revenue accuracy. If the receipt isn’t right, you’ve lost the retailer’s money and the shopper’s trust.
There are a dozen teams who predict that their prototypes are almost ready to handle the open-domain of retrofit stores and reach a revenue accuracy in the hard-range. They must also scale up to commercial size and operate continuously with 24×7 reliability. Despite the claims, most remain stuck in the proof-of-concept stage, having underestimated the OpenHard challenges lurking in the last mile of development. Grabango is the only company so far to have produced an OpenHard solution that works today in the real world. The shift from demonstration to repeatable business is a subtle one to see from the outside. Our systems are performing well at understanding the full domain of products, shoppers, and interactions in live, retail environments.
Amazon Go/JWO chose to drastically narrow the domain by constraining the store environment to the point where their tech would work. The Just-Walk-Out stores have a small number of SKUs that turnover very rarely and are carefully placed in just the right positions on special scales. Even minor rearrangements cause accuracy to plummet. This means that the JWO stores must have people continually curating the merchandise in service of the machine. Ironically, the JWO technology is a step backwards in automation as more labor is required to operate it than the 20th century stores it aims to replace. While the JWO concept performs very well for shoppers, the practical economics don’t work as a business. They retreated from the OpenHard challenge and settled instead for solving a far narrower problem; and in doing so, have so far surrendered the economics of a viable business.
To be applicable in the larger market for retrofits, Grabango chose the more difficult path of pursuing a generalized solution to the OpenHard problem. This means we resisted the many temptations to narrow the domain and instead elevated our technology to meet the wide open demands of the real world. We drove down the cost of hardware, developed a robust algorithmic approach, radically reduced computational requirements, deployed everything on distributed edge processing, and trained the AI until it delivered the exceptional hard-range accuracy that’s required. Finally, we protected the system with dozens of patent filings, entirely authored by our own team.
As of this writing, Grabango has deployed over 60,000 square feet of shopping floor, operates on 150,000 SKUs, and processes an incredible 22 petabytes of data every day. Across all stores, none have ever closed for installation or maintenance. Grabango’s revenue accuracy is presently 99.6%, as we march toward the company goal of 99.9%, far better than the 98.1% accuracy of legacy POS systems.
Grabango is the first organization to successfully operate checkout-free retrofits in the real world. We are thrilled to receive our industry’s AI Breakthrough Award for the Best Overall Computer Vision Solution. The award is in recognition of our OpenHard solutions in the open-domain of input type and hard-range of output accuracy. These OpenHard problems make up some of the most ambitious challenges in AI today and represent a meaningful step forward on the road toward AGI.